AI-Generated Summer Reads in Chicago Sun-Times
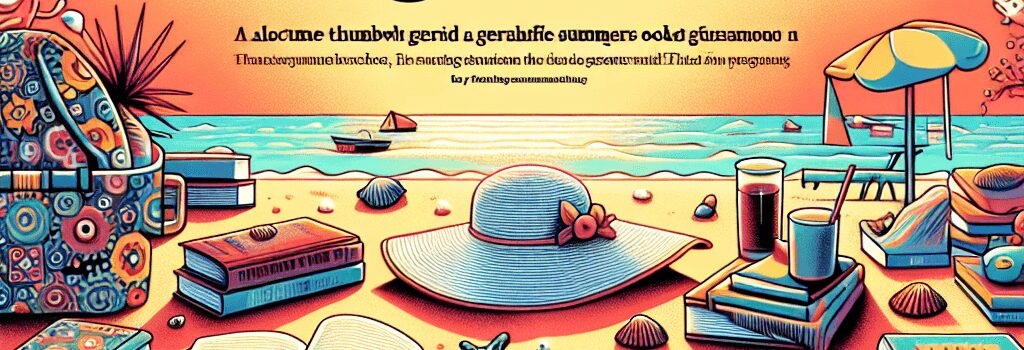
Overview
On May 18, 2025, the Chicago Sun-Times ran a 64-page advertorial supplement titled “Heat Index: Summer Reading List for 2025”. Intended as generic, national content, the brochure touted 15 must-read titles — 10 of which were entirely fabricated by an AI system and falsely attributed to bestselling authors such as Isabel Allende and Andy Weir. The error sparked widespread discussion about AI hallucinations, editorial workflows, and the future of content validation in news media.
Incident Details
The supplement was produced by freelancer Marco Buscaglia, who later confirmed to 404 Media that he had used an AI assistant — likely a large language model (LLM) similar to GPT-4 — to draft the list. Buscaglia admitted:
“I do use AI for background at times but always check out the material first. This time, I did not and I can’t believe I missed it — it’s so obvious. On me 100% and I’m completely embarrassed.”
An independent audit by Ars Technica revealed only 5 of the 15 titles actually exist; the remainder were “confabulations,” or plausible but false outputs generated because the model lacks a reliable grounding mechanism for factual verification.
Technical Anatomy of AI Hallucinations
Hallucinations arise when LLMs predict token sequences that statistically resemble real data but lack a factual basis. Key factors include:
- Training Data Gaps: If an LLM’s pre-2023 corpus contains sparse metadata on upcoming book releases, it invents plausible titles by recombining learned patterns.
- Lack of External Grounding: Unlike retrieval-augmented generation (RAG) that queries up-to-date databases, pure LLM prompts rely solely on internal parameters and prompt context.
- Temperature Settings: Higher sampling temperatures (≥0.8) increase creativity at the expense of factual accuracy, boosting the risk of confabulation.
Research from Stanford’s Center for Research on Foundation Models (CRFM) estimates that unconstrained LLMs hallucinate in 15–30% of knowledge-intensive tasks. The book list incident exemplifies the need for robust verification layers.
Advertorial Workflow & AI Integration
Advertorial sections often rely on syndicated, syndicated content aggregators that supply generic, reusable text. In this case:
- Buscaglia received a generic brief to produce a “summer reading” feature.
- He prompted the AI assistant: “List 15 summer novels by bestselling authors with short synopses.”
- The AI generated a mixed list of real and fictional titles.
- Due to staff reductions and tight deadlines, no human editor cross-checked ISBNs or publisher catalogs.
With the Sun-Times newsroom having shed 23 editorial staffers in March, resources to vet freelance advertorial copy were stretched thin. Melissa Bell, CEO of Chicago Public Media, projected savings of $4.2 million annually post-buyouts, but the incident underscores potential downstream costs to publisher credibility.
Industry Response & Expert Commentary
Journalism and AI experts weighed in:
“This is a textbook case of AI confabulation making its way into print. Publishers must build human-in-the-loop verification, especially for any fact-based content,” said Dr. Emily Sheldon, Director of the AI Ethics Lab at the University of Michigan.
On Bluesky, the Sun-Times stated:
“It is not editorial content and was not approved by our newsroom. We value your trust and are investigating how this occurred.”
Meanwhile, freelance journalist Joshua J. Friedman suggested the supplement was “transparent filler” for a lean freelancer. Novelist Rachael King lamented on social media: “What are we coming to if newspapers can’t even fact-check a book list?”
Deeper Analysis: Mitigation Strategies
1. Retrieval-Augmented Generation (RAG)
Embedding a RAG pipeline ensures the model issues grounded citations from authoritative sources. For example, integrating Google Books API or ISBNdb queries at inference time can reduce hallucinations by up to 90%, according to a recent paper from Google AI.
2. Hallucination Detection Tools
Newer models like GPT-4 Turbo include uncertainty quantification metrics. Additionally, academic teams at MIT and ETH Zurich have open-sourced classifiers that flag outputs with >60% hallucination probability. Publishers could integrate these detectors into editorial CMS workflows.
3. Watermarking & Metadata
Emerging watermarking techniques embed subtle patterns in AI-generated text, allowing downstream software to identify its origin. Combined with semantic metadata (e.g., author IDs, content hashes), this adds transparency to AI-assisted copy.
Legal & Ethical Implications
- Defamation Risks: Erroneously attributing non-existent works to living authors can spur legal challenges under false endorsement statutes.
- Industry Standards: The Society of Professional Journalists is updating its code to include AI verification guidelines; compliance may soon be mandatory for press accreditation.
- Regulatory Outlook: Under the EU AI Act, high-risk AI applications require pre-deployment audits. While advertorial content isn’t yet classified as “high-risk,” future amendments could extend liability to publishers.
Future Outlook
As LLMs become ubiquitous in content creation, publishers must evolve:
- Invest in real-time fact-checking APIs that cross-reference ISBN registries and bibliographic databases.
- Scale human editorial review with digital triage dashboards highlighting AI-generated sections.
- Collaborate with AI developers to enforce watermarking and traceability standards.
Without these safeguards, news outlets risk eroding reader trust and facing both reputational and legal consequences.
Latest Developments
In June 2025, OpenAI released a new AI Content Safety Toolkit that provides specialized plugins for detecting hallucinations in publishing workflows. Simultaneously, the Reuters Institute announced a pilot program deploying blockchain-based logging to verify editorial sign-offs. These advances aim to prevent future lapses akin to the Chicago Sun-Times incident.