AI Overhaul at HUD by College Student Under Musk’s DOGE
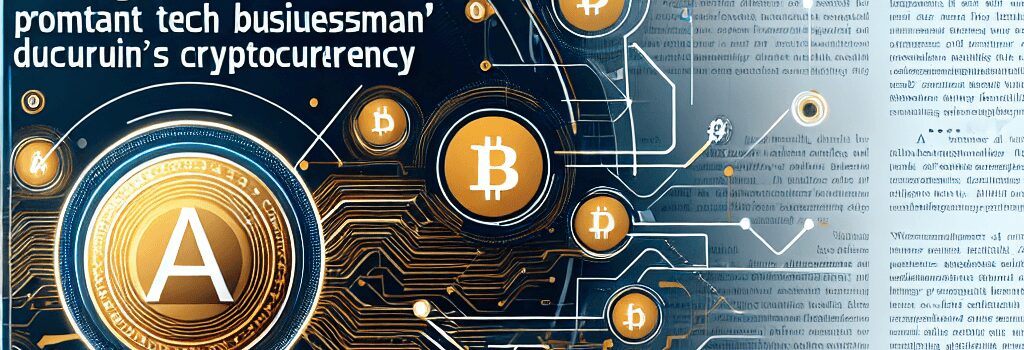
Introduction
In an unprecedented move blending private-sector AI innovation and federal rulemaking, Elon Musk’s Department of Government Efficiency (DOGE) has embedded a 20-year-old University of Chicago junior, Christopher Sweet, within the U.S. Department of Housing and Urban Development (HUD). Tasked with using advanced machine learning to audit, revise, and potentially eliminate hundreds of HUD regulations, Sweet’s role highlights both the promise and perils of “industrial-scale deregulation.”
Program Overview
Sweet joined HUD in early April 2025 as a “special assistant” to the HUD DOGE team. Despite lacking formal government experience, he has been granted read access to HUD’s Public and Indian Housing Information Center (PIHIC) and enterprise income verification systems. His mandate: leverage AI to compare existing regulations against statutory laws, pinpoint “overreach,” and propose streamlined replacement language.
- Source systems: PIHIC database, Enterprise Income Verification (EIV) interface, eCFR corpus.
- Deliverables: Spreadsheets containing ~1,200 flagged regulatory clauses, quantified compliance scores, and AI-generated rewrite suggestions.
- Workflow: PIH subject-matter experts review, annotate, and either accept or contest AI recommendations; final drafts are routed to HUD’s Office of the General Counsel.
Technical Underpinnings of the AI Model
The AI engine driving Sweet’s project is reportedly a fine-tuned large language model (LLM) based on an open-source Transformer architecture with approximately 20–30 billion parameters. Key technical features include:
- Pretraining Corpus: Full text of the Electronic Code of Federal Regulations (eCFR), Federal Register archives, and comparative state statutes.
- Fine-Tuning: Supervised learning using a curated dataset of 10,000 annotated regulation-statute pairs to teach the model legal alignment and policy simplification.
- Prompt Engineering: Dynamic templates that extract context windows of up to 8,192 tokens, enabling cross-referencing of statutory mandates and existing rule text in a single query.
- Evaluation Metrics: BLEU and ROUGE scores for textual fidelity, plus bespoke “regulatory compliance ratios” derived from a rule-based parser that counts conditional clauses and mandatory language constructs.
Legal and Procedural Implications
Under the Administrative Procedure Act (APA), federal agencies must undergo multi-stage notice-and-comment periods and justify all significant rule changes. Critics argue that deploying AI to preemptively draft regulatory language could circumvent essential public-feedback loops. Legal experts warn of several potential pitfalls:
- Lack of transparency: AI decision logic may be opaque, hindering judicial review.
- Rule validity: Courts may strike down regulations if AI-based justifications aren’t accompanied by adequate human deliberation.
- Data governance: Integrating AI outputs into official Federal Register notices requires strict chain-of-custody documentation.
Security and Privacy Risks
Granting AI systems access to HUD’s sensitive databases raises cybersecurity and privacy concerns. Government officials and external auditors have flagged the following risks:
- Cloud Infrastructure: The AI runs on a hybrid cloud environment using AWS GovCloud, requiring FedRAMP Moderate authorization. Misconfigurations could expose PII of public-housing residents.
- Access Control: Role-based access control (RBAC) must be strictly enforced. Unvetted AI prompts could inadvertently query confidential case files.
- Model Drift: Continuous retraining on live data can introduce biases or drift, necessitating routine evaluations against a secure validation set.
Expert Opinions
Dr. Anita Rao, a regulatory scholar at Georgetown University, notes: “AI can accelerate draft revisions, but real regulatory wisdom arises from stakeholder engagement and impact analyses—elements that no algorithm can fully replicate.” Meanwhile, cybersecurity specialist Marcus Chen from the Center for Internet Security warns, “Embedding unproven AI pipelines into mission-critical systems without thorough penetration testing contravenes federal security best practices.”
Broader Context and Next Steps
Sweet’s work is part of the Trump-era Project 2025 blueprint, which many ex-officials now implement across agencies to pare back regulations in areas like environmental protection, FDA oversight, and diversity initiatives. According to recent Office of Management and Budget (OMB) guidance, agencies must now include AI impact statements in all deregulatory rulemaking packages.
In the coming weeks, HUD staff will finalize an AI-augmented draft of PIH regulations, submit it to the General Counsel, and prepare it for public comment. Simultaneously, the refined AI model is slated for rollout at the Departments of Education and Energy later this year.
Conclusion
The integration of AI into federal regulatory processes marks a transformative moment in public administration. Yet it also prompts urgent questions about transparency, accountability, and the preservation of democratic safeguards. As Christopher Sweet leads this high-profile experiment at HUD, policymakers and technologists will be watching closely to see if AI-driven deregulation can maintain both efficiency and legitimacy in the rule-making process.