Social Media Use Increases Susceptibility to Fake News
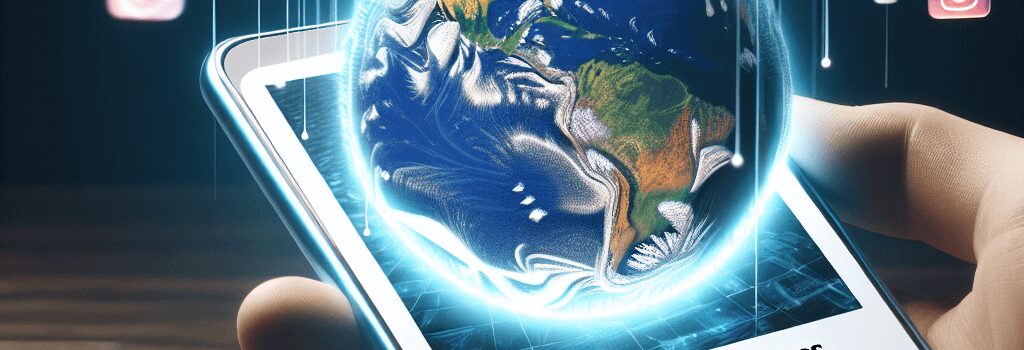
As social platforms become central to daily life, researchers are uncovering how problematic social media use (PSMU)—a pattern of engagement sharing parallels with behavioral addictions—can amplify the spread and belief in fake news. A new PLoS ONE paper by Dar Meshi and Maria Molina at Michigan State University not only quantifies this link but opens avenues for technical and policy solutions in 2025’s evolving media landscape.
Understanding Problematic Social Media Use (PSMU)
PSMU is characterized by six core biopsychological components of addiction, adapted from substance-use criteria. Although PSMU is not yet in the DSM-5, its assessment parallels established scales like the Bergen Social Media Addiction Scale (BSMAS). In their study, Meshi and Molina operationalized PSMU via self-report items probing:
- Withdrawal symptoms (e.g., anxiety when unable to check feeds)
- Tolerance (needing increasing screen time for the same ‘reward’)
- Relapse (unsuccessfully attempting to cut down usage)
- Salience (preoccupation with notifications and posts)
- Mood modification (using social media to alleviate stress)
- Conflict and impairment (social, academic or occupational consequences)
By anchoring to these six dimensions rather than raw time-on-site metrics, the researchers ensured their PSMU index reflected functional impairment—a critical distinction echoed in the latest International Classification of Diseases (ICD-11) guidelines under review.
Study Design and Technical Specifications
Meshi and Molina recruited 189 college students, balanced for age and field of study, via a secure Qualtrics survey. Participants completed:
- A 24-item PSMU questionnaire (Cronbach’s α = 0.87)
- An 8-item Impulsivity Scale (Barratt Impulsiveness Scale v11)
- An attention-check battery to filter inattentive responders
Subjects then evaluated 20 social-media-style posts (10 real, 10 fabricated) formatted to mimic Twitter’s X and Facebook’s News Feed interfaces. Custom JavaScript recorded latencies for each action—click, like, share, comment—providing millisecond-level granularity. Participants rated perceived accuracy (1–7 Likert scale) and engagement likelihood via calibrated UI elements built with React.js.
Key Findings
Data analysis using Python’s SciPy package and R’s lme4 revealed that higher PSMU scores correlated with:
- Increased accuracy judgments for fake items (r=0.42, p<0.001)
- Higher click-through rates on misinformation posts (mean 37% vs. 15% in low-PSMU group)
- Greater self-reported likelihood to share or comment on false content (OR=2.3, 95% CI [1.5–3.6])
Importantly, genuine news engagement also rose with PSMU, indicating a broader impulsivity-driven interaction style rather than mere gullibility.
Neural Mechanisms Underlying PSMU and Misinformation Susceptibility
Meshi, a neuroscientist by training, hypothesizes that PSMU involves dysregulated dopaminergic signaling in the mesolimbic pathway—particularly the ventral tegmental area (VTA) to ventral striatum projections. Functional MRI studies (e.g., an April 2025 University of Pennsylvania paper) have shown reduced prefrontal cortex (PFC) engagement during risk evaluation in high-PSMU individuals, impairing inhibitory control and reward discounting.
“When reward prediction errors fire up in the striatum, a user may interpret sensational headlines as more valuable than their real risk,” explains Dr. Elena Rodriguez, a cognitive neuroscientist at MIT. “Over time, synaptic plasticity in the nucleus accumbens may reinforce superficial engagement patterns, making fake content harder to resist.”
Implications for Platform Design and Moderation
In late 2024, Meta rolled out an AI-powered rumor classifier employing transformer-based models (e.g., RoBERTa, BERT variants) to flag high-risk posts. Preliminary A/B tests showed a 12% reduction in virality of flagged content among users with high engagement scores but have not yet addressed PSMU-specific vulnerabilities.
Twitter’s 2025 pilot of generative-AI fact-check labels—for which early metrics demonstrate a 5% bump in correction clicks—also offers a promising countermeasure. However, experts caution that UX friction must be balanced: excessive pop-ups may exacerbate withdrawal in problematic users, triggering relapse-like behaviors.
Future Directions: Monitoring, Intervention, and AI Integration
Building on Meshi and Molina’s groundwork, next steps include multimodal monitoring combining behavioral logs, ecological momentary assessment (EMA), and wearable data (heart-rate variability as a stress proxy). AI-driven digital phenotyping could identify PSMU red flags in real time, enabling just-in-time interventions (JITI) such as micro-break prompts.
Therapists and digital health startups are exploring chatbot agents powered by large-language models (e.g., GPT-4 Turbo) to deliver cognitive-behavioral strategies when a user’s engagement patterns align with PSMU profiles. Early trials at Stanford’s Center for Digital Health show a 20% decrease in self-reported withdrawal symptoms over eight weeks.
Conclusions and Expert Recommendations
Meshi emphasizes moderation, not eradication: “Social media offers genuine social capital, especially for isolated demographics. Like alcohol, its benefits turn detrimental without self-regulation.” He advocates for platform APIs to expose aggregated PSMU metrics to researchers under strict privacy protocols.
As disinformation threats evolve, combining neuroscience insights, robust UX design, and AI-driven moderation will be critical to mitigating PSMU-driven misinformation proliferation. Stakeholders—from developers to policymakers—must collaborate to create healthier digital ecosystems in 2025 and beyond.