Quantum Hardware: Paving the Way for AI’s Next Quantum Leap
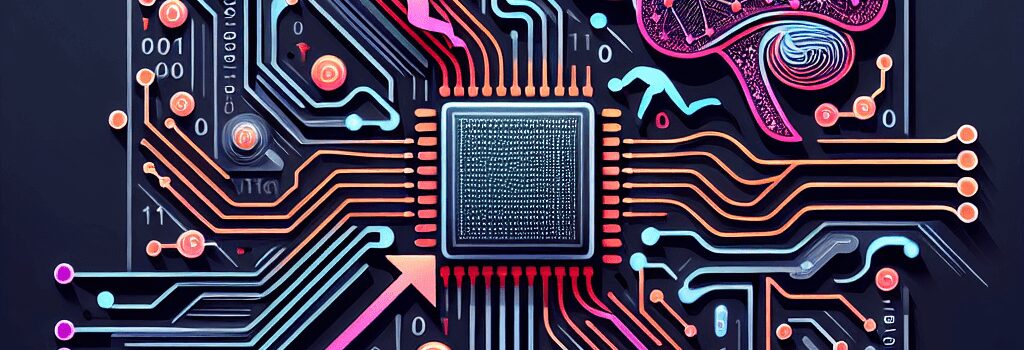
Introduction
As AI models continue to push the boundaries of capability, concerns about their energy consumption and processing efficiency are driving researchers to seek novel hardware solutions. One promising frontier is quantum computing. Although in its nascent stage, quantum hardware offers a fundamentally different architecture that naturally aligns with some of the mathematical challenges underlying machine learning and neural networks.
Quantum Computing Meets Machine Learning
At its core, quantum computing leverages qubits and quantum gates that allow for inherently parallel computations. Unlike traditional systems that separate processing from memory, quantum systems store data directly in qubits, thereby reducing the memory bottleneck associated with classical computing. This architecture may provide significant advantages when executing complex matrix operations often required in deep learning algorithms.
Recent research illustrates this synergy through the use of variational quantum circuits, where two-qubit gate operations mimic the communication between artificial neurons. In this approach, additional parameters—akin to neural network weights—are fed through classical control signals that shape the behavior of the quantum circuit. Such integration of classical control with quantum operations provides a compelling pathway for future AI algorithms.
Practical Applications: From Pixels to Qubits
One of the latest studies, a collaborative effort involving the Honda Research Institute and the quantum software company Blue Qubit, focused on translating traditional image data into a quantum framework. Utilizing the Honda Scenes dataset, which comprises images captured during 80 hours of driving in Northern California, the team challenged their system to detect a simple but pertinent feature: whether it was snowing in the scene.
To process these images, the researchers applied three different encoding strategies to convert pixel data into quantum information. These approaches varied based on how the image was segmented and the number of qubits assigned to each segment, thereby affecting the resolution and overall performance of the classification task. The training phase was conducted on a classical simulator to determine the optimal parameters, analogous to setting the weights in a neural network. The tuned operations were then executed on two different quantum processors—one from IBM featuring 156 qubits with a comparatively higher gate error rate, and one from Quantinuum, which, despite having only 56 qubits, delivered a very low error rate during operations.
Technical Deep Dive and Performance Insights
While the classification accuracy achieved by the quantum processors was above random chance, it still lagged behind state-of-the-art classical algorithms. However, the significance of these experiments lies in the demonstration that real-world quantum hardware is progressively capable of running AI models. As gate error rates decrease and qubit counts increase through technological improvements, quantum systems could ultimately surpass classical systems in specific machine learning tasks.
- Processor Specifications: IBM’s quantum processor uses a large array of 156 qubits, providing greater potential for parallelism but struggling with higher noise levels. In contrast, Quantinuum’s processor, with its 56 qubits, delivers a more stable performance due to its lower gate-error rate.
- Data Encoding Methods: The study analyzed multiple methods of encoding classical image data into quantum systems, each with different trade-offs between qubit utilization and the fidelity of feature extraction.
- Variational Quantum Circuits: The use of two-qubit gate operations in conjunction with classical control parameters opens a pathway to emulate traditional neural network interactions on quantum hardware.
Analyzing the Hardware Challenges
Running AI algorithms on quantum processors is not without its challenges. The current quantum hardware still faces significant hurdles in terms of error correction, qubit coherence times, and scalability. For instance, while increasing the number of qubits can improve the representation of data and potential parallel processing, it also introduces compounded error rates that can compromise the integrity of computations. Maintaining a delicate balance between qubit count and operational efficiency is the foremost challenge researchers are attempting to overcome.
Experts in the field argue that innovations in error mitigation and fault-tolerant quantum computing are crucial for realizing practical quantum AI. Dr. Elena Martinez, a quantum computing researcher at a leading tech institute, remarks, “We are entering an era where the hybridization of classical and quantum approaches will likely yield breakthroughs that neither technology could achieve alone. The lessons learned from early experiments are invaluable in steering the next generation of hardware improvements.”
Future Directions and Expert Opinions
Despite being in its early stages, the fusion of quantum computing with AI presents exciting opportunities. Beyond image classification, future research is looking to apply quantum-enhanced algorithms to natural language processing, optimization problems, and even reinforcement learning environments. Researchers anticipate that as quantum hardware matures, we will see a shift from feasibility studies to real-world applications involving complex datasets and dynamic environments.
Industry leaders and startups alike are investing in hybrid architectures that couple classical supercomputers with quantum accelerators. This approach is seen as a promising interim step, enabling the best of both worlds—leveraging quantum speed-ups for select subroutines while maintaining the robustness of classical computation for the overall task orchestration.
Conclusion
While modern quantum hardware is still on the cusp of operational excellence for AI applications, the recent advancements underscore its potential. The collaborative efforts to pull classical data into the quantum realm through innovative encoding and variational circuits have laid the groundwork for future machine learning breakthroughs. As error rates drop and qubit counts soar, the intersection of quantum computing and AI could redefine computational paradigms, driving us towards a future of more efficient and powerful machine learning processes.
In summary, although quantum AI still faces technical challenges, ongoing research and experimental validations are fueling optimism that these hurdles will be overcome. With continued innovation and closer collaboration between academia and industry, quantum-enhanced AI may soon transition from theoretical possibility to practical reality.
Source: Ars Technica