Outsourcing Confirmation Biases: How Search Engines Shape Our Perceptions
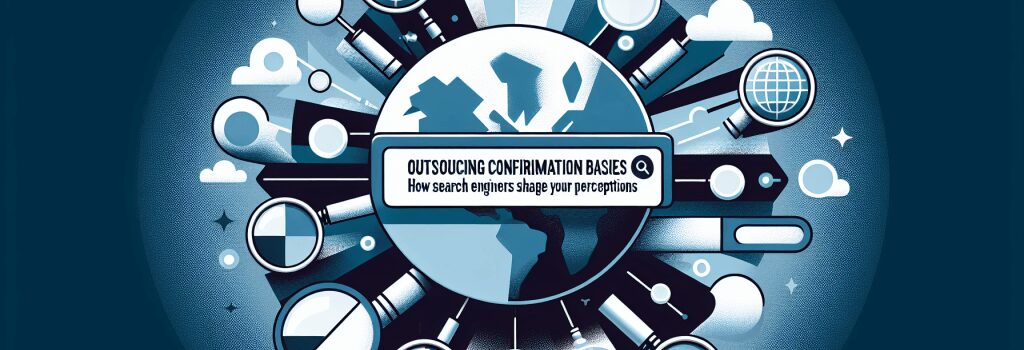
Our digital landscape is increasingly defined by the way search engines present information. While users often shape their queries to align with their pre-existing beliefs, recent research suggests that small tweaks—from adjusting query phrases to reformulating search results—can have a profound impact on how opinions evolve. This article delves into the technical details behind these findings, examining both human behavior and algorithmic responses, and considers the opportunities and pitfalls of re-engineering search experiences.
The Role of Search Engines in Reinforcing Biases
The modern search engine is more than a repository of links; it is an active gatekeeper of information. As demonstrated in studies by Tulane University’s Eugina Leung and the University of Chicago’s Oleg Urminsky, individuals routinely formulate narrow search queries that tend to return results confirming their existing views. By typing queries such as ‘Is caffeine bad for you?’ rather than ‘What are the health effects of caffeine?’, users inadvertently narrow the spectrum of data available to them.
Using tools like Google Adwords Planner, the researchers evidenced that more than a quarter of caffeine-related search queries are highly focused, emphasizing selective data retrieval rather than a balanced overview. This tactical bias reinforces what is known as confirmation bias—the human tendency to favor information that confirms one’s preconceptions.
Technical Insights: Algorithms and Query Manipulation
Technically, search engine algorithms are designed to match query keywords with indexed content. However, as search engines rely heavily on keyword relevance and historical data, the specificity of a query can dictate a skewed or partial dataset. Developers have tried to counteract this by refining ranking signals to emphasize reputable sources. Nonetheless, as algorithms get refined, savvy content creators often learn to game them, introducing a continuous cat-and-mouse dynamic between algorithm updates and user behavior.
Recent innovations in AI and machine learning have started to address some of these challenges. Advanced natural language processing models are now capable of interpreting context and sentiment, making it more feasible to detect and counteract overly specific, biased queries without compromising the user experience. For example, the AI-powered version of Bing search has been observed reformulating queries to generate more balanced search results, indicating a significant step towards algorithmic neutrality.
Empirical Study: Searching for Affirmation
The study at the center of this discussion used controlled experiments where participants were asked opinion-based questions—such as, ‘Is caffeine beneficial or harmful?’—and then directed to search online for evidence supporting or refuting this view. The findings were consistent: when participants crafted biased, narrowly focused queries, they were more inclined to retain their original opinions, supported by results that merely echoed their beliefs.
Analysis of Google Trends data further reinforces this notion. The research highlights regional biases, for instance, illustrating that states with a higher Republican vote share were more likely to generate queries like ‘Trump won’ over more neutrally phrased searches. This linkage between cognitive bias and search behavior underscores the importance of query strategy in shaping public opinion.
Algorithmic Manipulation and Ethical Considerations
Additional experiments conducted by the researchers experimented with algorithmic intervention. In one set of tests, all participants received identical, broad-spectrum results irrespective of their search terms. This approach significantly increased the likelihood that participants’ opinions would shift, as they were exposed to a wider range of information. This raises intriguing ethical and technical questions: How much should algorithms intervene in tailoring search results?
- Transparency: Developers must ensure that any modifications to search algorithms are transparently communicated to avoid accusations of censorship or manipulation.
- Quality vs. Quantity: Balancing the importance of presenting a broad spectrum of information without inundating users with irrelevant data is a delicate task that requires robust quality metrics.
- User Autonomy: There is a tension between nudging users towards diverse perspectives and preserving their autonomy. The ethical implications of algorithmic bias reduction need thorough scrutiny from both technical and sociopolitical standpoints.
Data-Driven Insights and Technical Perspectives
From a technical standpoint, the interplay between user-generated search terms and algorithmic filtering provides valuable insight into the design of intelligent systems. Machine learning algorithms must continue adapting to external manipulations and emergent patterns of user behavior. Future development efforts are likely to incorporate reinforcement learning techniques to dynamically adjust the weightage given to niche versus broad search terms, ensuring more balanced outcomes.
Furthermore, employing statistical models to detect patterns indicative of bias may help in creating safeguards against misinformation. By leveraging AI models that monitor and adjust for cognitive biases, search engines can evolve into platforms that not only provide information but also encourage critical thinking and informed decision-making.
Future Directions: AI, Machine Learning, and Search Engine Optimization
Looking forward, the potential for AI and machine learning to reshape search engine functionality is immense. Research continues in refining algorithms to not just serve relevant results, but to also detect and mitigate cognitive biases. Hybrid models that merge human oversight with AI adjustments could pave the way for search systems that promote belief updating with minimal intrusion.
Moreover, advancements in cloud computing infrastructure are enabling these sophisticated models to run at scale, facilitating real-time analysis of billions of queries. As researchers expand experiments into other domains—ranging from cryptocurrency trends to environmental policies—the lessons learned hint at broader applications for enhancing digital literacy and combating echo chambers online.
Conclusion
In summary, while search engines have long been partners in our quest for knowledge, their role in reinforcing or challenging our biases depends critically on both user behavior and technological design. By recognizing the impact of narrowly tailored search queries and deploying AI-based enhancements, we can foster a digital ecosystem where information serves as a bridge rather than a barrier to open-mindedness. These developments remain vital as we continue to navigate the complex interplay of human cognition and algorithmic influence in our information-rich era.