Can Law Keep Up with Climate Science and Damages?
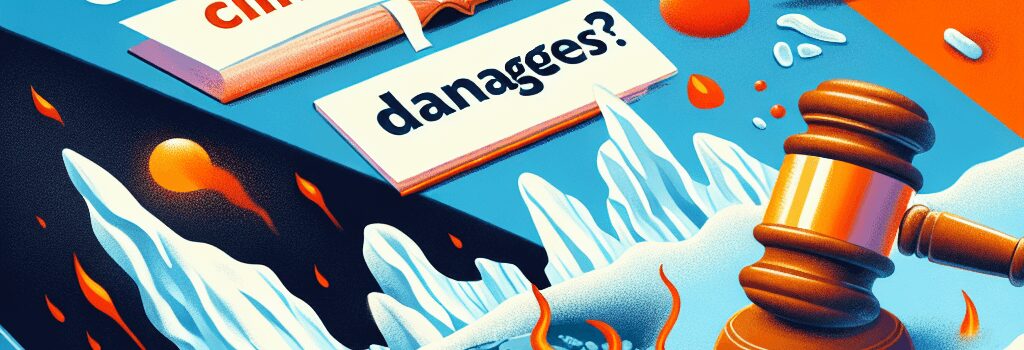
Attribution science has matured rapidly over the past decade. Where once it was impossible to link a single weather event to anthropogenic warming, researchers can now trace the human fingerprint on heat waves, hurricanes, and wildfires in near real time. Dartmouth climatologists Christopher Callahan and Justin Mankin have extended this rigor to economics, estimating that emissions from the world’s 111 “carbon majors” have generated roughly $28 trillion in cumulative damages through 2020. Their peer-reviewed methodology not only refines our understanding of climate impacts but also offers a concrete “but-for” framework that could anchor future legal claims against fossil fuel companies.
How Attribution Science Has Evolved
Traditional event attribution compares two ensembles of climate simulations—one reflecting observed greenhouse gas concentrations, the other a counterfactual world without anthropogenic CO₂—using state-of-the-art global climate models (GCMs) such as those in the CMIP6 suite. Advances in high-resolution dynamically downscaled models (e.g., convection-permitting simulations at 1–4 km grid spacing) and machine-learning surrogates now allow for rapid, precise estimates of how warming alters the probability and severity of extremes. The World Weather Attribution consortium and the IPCC’s AR6 detection chapter have formalized these approaches, providing robust statistical frameworks and open-source tools for global research teams.
Company-Level Emissions and Temperature Increments
Callahan and Mankin’s innovation lies in apportioning global warming to individual firms by integrating company-specific emission inventories—both Scope 1 (production) and Scope 3 (combustion)—with an impulse-response climate model like FAIR or MAGICC. By scaling the total CO₂ forcing down to each “carbon major,” they calculate the company-attributable fraction of the ~1.2 °C global temperature increase since pre-industrial times. For example, ExxonMobil’s emissions after 1990 account for roughly 0.017 °C of warming in 2020. These fine-scale forcings feed into ensembles that simulate extreme temperature distributions with and without the company’s emissions.
Translating Temperature Increments to Economic Costs
Linking temperature anomalies to financial losses relies on econometric damage functions, which draw on country-level panel datasets of GDP, agricultural yields, mortality rates, and infrastructure repair costs. Recent peer-reviewed studies estimate a nonlinear β coefficient between daily maximum temperature and per-capita income growth, often parameterized as a piecewise function with thresholds above 29 °C triggering steep declines in labor productivity and crop output. Callahan and Mankin apply these functions to the simulated extreme-heat days attributable to each company, yielding per-year cost estimates that factor in regional vulnerability indices and adaptive capacity.
Massive Global Economic Damages
Running thousands of Monte Carlo trials, the team derives probabilistic damage ranges. Chevron’s cumulative liability from 1991–2020 spans $0.8–3.6 trillion, with a median estimate near $1.9 trillion. Saudi Aramco, Gazprom, ExxonMobil, and BP follow closely, each ranked by their emissions share and regional exposure. Summing across all 111 entities yields a central estimate of $28 trillion in lost economic output—an amount comparable to the annual GDP of the U.S. and China combined.
Technological Advances in Model Resolution and AI-Enhanced Attribution
Next-generation attribution leverages AI to downscale GCM outputs via neural networks trained on high-resolution regional climate models. Platforms like NASA’s Earth Exchange (NEX) and ECMWF’s Copernicus Climate Cloud provide petascale HPC resources for ensemble runs exceeding 1,000 members. Novel techniques such as Physics-Informed Neural Networks (PINNs) enable real-time bias correction and probabilistic forecasting, cutting end-to-end analysis times from months to days. These innovations promise even finer company-level liability mapping, potentially at the level of individual oil fields or coal mines.
Regulatory and Legal Frameworks
Courts typically require plaintiffs to meet a “but-for” causation standard. The quantitative rigor of modern attribution aligns precisely with this legal test. In Ryan v. the State of Montana, youth plaintiffs argued that state policy violated constitutional rights to a clean environment, citing scientific evidence of health impacts. In Europe, Lliuya v. RWE marked the first insurer to quantify a flood risk attributable to a single energy firm. In the U.S., dozens of municipalities have filed public nuisance suits, while investors pursue securities-fraud cases alleging climate risk misrepresentation under SEC rules.
Economic Uncertainties and Risk Quantification
Despite methodological advances, uncertainty remains in damage-function parameterization, socioeconomic projections, and adaptive responses. Further research is needed to standardize uncertainty quantification—through value-at-risk (VaR) and expected shortfall metrics used in financial risk management—and to develop guidelines for admissible evidence in court. Cross-disciplinary collaboration between climatologists, economists, and legal scholars will be crucial to refine defensible liability models.
Future Directions and Policy Implications
The fusion of high-resolution climate modeling, econometric impact assessment, and AI-driven analysis is forming a new backbone for climate litigation. As attribution frameworks become more transparent and accessible, we can expect a surge of lawsuits aimed at recouping societal costs and forcing corporate accountability. Policymakers may soon integrate these quantified liabilities into carbon pricing schemes, sovereign debt assessments, and transition risk regulations. In this emerging landscape, the law may finally catch up with the science—and ultimately the scale of the crisis itself.
Reference: Callahan, C. & Mankin, J. (2025). Monetized economic damages from individual carbon majors. Nature. DOI: 10.1038/s41586-025-08751-3.