AI for AI Safety: Harnessing Frontier AI to Protect Our Future
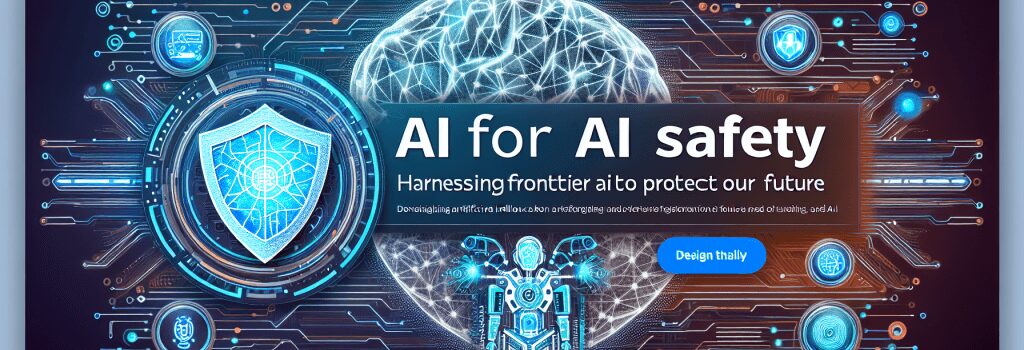
Published on March 14, 2025 3:00 PM GMT
(Audio version here (read by the author), or search for “Joe Carlsmith Audio” on your podcast app.)
This is the fourth essay in a series called “How do we solve the alignment problem?” For a series introduction and a summary of the previous essays, please see this introduction.
In the previous essay, I introduced a high-level framework for visualizing the journey towards safe superintelligence. This framework was underpinned by three pivotal “security factors”:
- Safety progress: Our ability to incrementally develop advanced AI capabilities in a reliable and secure manner.
- Risk evaluation: The tools and methodologies we employ to assess potential dangers associated with specific AI developments.
- Capability restraint: The mechanisms that enable us to guide and moderate AI progress in situations where uncontrolled advancement could lead to catastrophic risks.
This essay further argues for the essential role of what I call “AI for AI safety.” This approach leverages frontier AI labor to reinforce the above security factors. It involves two interconnected feedback loops:
- The AI capabilities feedback loop: Where access to rapidly improving AI systems accelerates further advancements in AI capability.
- The AI safety feedback loop: Where controlled and secure access to frontier AI technology is used to enhance safety measures and risk evaluation methods.
In essence, deploying AI for AI safety means ensuring that the safety feedback loop either outpaces or constrains the raw capability feedback loop, thereby keeping us within a safe operational envelope.
2. Defining AI for AI Safety
By “AI for AI safety,” I refer to any strategy that leverages emerging AI capacities to boost our competence on the alignment problem without having to wait for breakthroughs solely driven by human labor. Below, I further break down this concept according to the major security factors.
2.1 Enhancing Safety Progress
A prominent application here is automated alignment research: using AI systems to ideate, test, and refine methods that constrain AI motivations and ensure compatibility with human interests. Advanced tools are already integrated into various alignment processes such as:
- Evaluating AI outputs to ensure they follow preset safety guidelines.
- Labeling neuronal activations for better mechanistic interpretability.
- Monitoring chain-of-thought processes to catch reward-hacking techniques.
- Classifying transcripts and identifying malicious or alignment-faking behaviors.
Looking ahead, the vision is for AI to eventually automate the full pipeline—generating novel ideas, conducting experiments with robust statistical methodologies, critiquing results, and quickly remediating alignment shortcomings. Indeed, some leading labs are investing heavily in this automation, which I believe could become the single most effective means of using AI labor for safety.
2.2 Strengthening Risk Evaluation
Automated evaluation systems are envisioned to design and conduct comprehensive risk assessments. Examples might include:
- Creating and managing complex evaluation pipelines autonomously.
- Running safety cases and cost-benefit analyses using sophisticated simulation models.
- Enhancing our scientific understanding of AI behavior through data-driven model organism experiments.
Through the integration of advanced forecasting algorithms and a refined collective epistemology, AI can provide quantitative feedback that significantly bolsters our risk evaluation frameworks.
2.3 Facilitating Capability Restraint
Capability restraint focuses on preventing undesirable forms of rapid capability escalation. AI-enabled systems can promote restraint by:
- Individual caution: Offering risk evaluations and counterfactual simulations that inform better decision-making by developers.
- Enhanced coordination: Acting as automated mediators or negotiators to facilitate mutually beneficial agreements and enforce commitment mechanisms.
- Restricted options and enforcement: Pioneering on-chip monitoring, advanced cybersecurity protocols, and legally binding policy designs to minimize risks associated with AI overreach.
Such technical measures can also be integrated with export control frameworks and even augmented into military applications, where necessary.
3. Dual Feedback Loops: Opportunity and Risk
The core of the AI for AI safety strategy lies in understanding two primary feedback systems. The first, the capability feedback loop, is driven by a rapidly iterating cycle where improvements in AI boost the capacity for further refinement and expansion. Under this process, both human and AI labor contribute, and as algorithms mature, the quality of AI labor itself improves.
In contrast, the safety feedback loop ensures that these improvements are implemented within a secure and controlled environment. In this diagrammatic view, AI assists in elevating the security factors that in turn allow us safe access to even more advanced AI tools. The objective is to dynamically adjust and extend the safety envelope—either by outpacing the capability loop or through deliberate restraint measures.
4. The AI for AI Safety Sweet Spot and Spicy Zone
The interplay of the feedback loops introduces the notion of an “AI for AI safety sweet spot.” This is a capability window where frontier AI systems have reached a point at which they can substantially improve our risk evaluation and restraint technologies, but remain far from being able to override human control. Conceptually, this zone offers a balanced trade-off:
- Advanced enough to catalyze breakthroughs in safety and capability restraint.
- Still under effective countermeasures that block any attempt by AI to disempower humanity.
A further extension of this concept is the “AI for AI safety spicy zone.” In this regime, systems are approaching conditions where their power is so substantial that ensuring they lack any options to disempower humanity requires robust motivation control. This zone is technically more challenging and demands heightened research on oversight and incentive design.
5. Contrasting Views: Human-Driven vs. AI-Driven Safety Approaches
Critics of AI for AI safety often argue for reliance on radical human-labor-driven alignment progress rather than automated AI-driven approaches. Proponents of the human-centric view assert that without enhancing human cognitive capacities (e.g., via whole brain emulation or advanced brain-computer interfaces), AI labor remains risky and unpredictable.
On the other hand, AI for AI safety emphasizes a more direct approach by harnessing the enormous computational and analytic power of frontier AIs directly. This method attempts to sidestep the lengthy process of human augmentation and, with proper countermeasures, directly channels AI effort into solving its own alignment challenges.
This debate continues to evolve as both approaches are supported by varying expert opinions and strategic forecasts. Notably, debates currently span the theoretical frameworks of differential technological development and practical implementations of on-chip monitoring and cybersecurity enhancements.
6. Deep Dive: Technical and Policy Implications
Beyond the high-level concepts, it is essential to explore the technical challenges and policy frameworks that must accompany AI for AI safety. Current research is focusing on:
- Automated Experimentation Pipelines: Developing systems where AI autonomously designs, runs, and analyzes experiments to test alignment hypotheses. For example, leveraging reinforcement learning and advanced simulation environments can reduce iteration time while increasing reliability.
- Formal Verification Methods: Integrating formal methods into AI systems to ensure robustness. Techniques such as model checking and proof assistants are now being refined to address safety properties in real-time code execution.
- Data Safety and Security Protocols: Given the risk of rogue actors exploiting vulnerabilities, policymakers and technologists are collaborating on standards for secure data exchange, with cryptographic methods like zero-knowledge proofs becoming increasingly relevant.
Experts in the field, including researchers from top AI labs and cybersecurity institutes, underscore the necessity of synchronizing regulatory policy with technical innovation. As AI systems become more autonomous, regulatory bodies are being advised to consider adaptive policy tools that evolve alongside technology advancements.
7. Expert Opinions and Industry Trends
Recent statements by leading figures in AI research have bolstered the case for AI-driven safety measures. For instance, a recent panel discussion at a global tech conference highlighted that:
- Advanced AI systems can now simulate complex scenarios that were once unthinkable a decade ago, making them invaluable for preemptive risk assessments.
- Industry leaders are increasingly advocating for dual-use AI frameworks, where the same technological advances serve both efficiency and safety purposes.
- Collaborations between academic researchers, regulatory bodies, and corporate entities are creating ecosystems that can rapidly prototype and iterate on safety protocols.
These developments mirror the rapid digital transformation witnessed in other domains like cloud computing and cybersecurity, where automated systems have historically outpaced human intervention. As such, dedicating resources to AI-driven alignment research is seen as both a timely and necessary advance in our technology ecosystem.
8. Future Perspectives and Policy Recommendations
Looking forward, several recommendations have emerged from both technical experts and policy advisors:
- Invest in Scalability: Support research initiatives that bridge the gap between human and AI labor in alignment, ensuring that safety measures scale alongside capability advancements.
- Foster Public-Private Partnerships: Encourage a collaborative approach between tech companies, academic institutions, and government agencies to create comprehensive oversight frameworks.
- Create Adaptive Regulations: Develop policies that are flexible enough to adapt to the rapid evolution of AI technology, particularly as systems approach or move beyond the sweet and spicy zones described above.
- Enhance Global Coordination: In an interconnected world, international standards and cross-border partnerships will be key to mitigating risks associated with AI overreach.
Maintaining a balance between innovation and control in AI research is not only a technical challenge but a socio-political one. As experts continue to navigate these transformative changes, policies must evolve to not only incentivize progress but also ensure the broadest possible safety net.
9. Conclusion and Actionable Steps
In summary, the strategic use of AI labor for improving AI safety presents an unprecedented opportunity to transform our approach to one of the most significant challenges of our time. By harnessing the dual feedback loops of capability and safety, we can, in principle, channel technological progress towards a future where even highly advanced AI systems remain under human control.
However, the path forward is complex. It demands a rigorous scientific approach combined with proactive policy-making. The steps outlined—from achieving the sweet spot, building robust countermeasures, to developing scalable oversight frameworks—offer a blueprint for leveraging frontier AI to secure a safer technological future.
As the landscape evolves, continued dialogue among AI researchers, cybersecurity experts, and policy makers will be crucial. Timely action, coupled with adaptive, technically informed regulation, will determine whether we can effectively harness AI for both innovation and safety.