METR Revisited: Unveiling the Metric of AI’s Autonomy in Tackling Extended Tasks
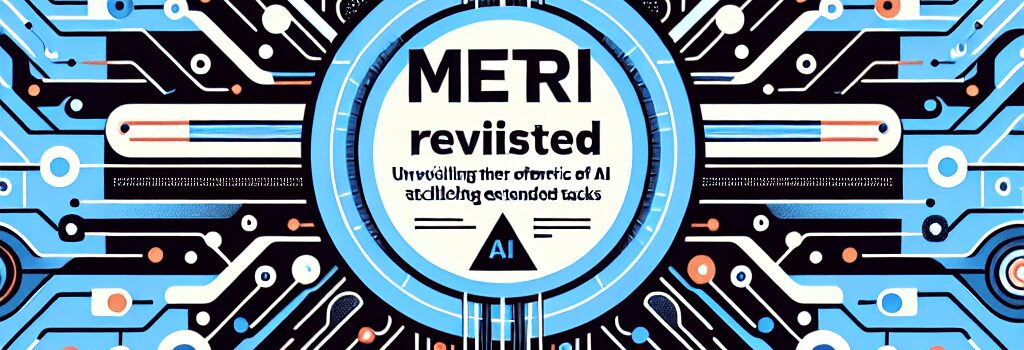
Published on: March 19, 2025 4:00 PM GMT
Summary: In this work we present a novel perspective on evaluating AI performance by assessing the length of tasks that AI systems can complete autonomously. Over the past six years, the capacity of these systems has improved exponentially, with the time horizon effectively doubling every 7 months. Extrapolating these trends indicates that, within the next few years, AI agents will be capable of handling complex, multi-step projects that currently require days or even weeks of human effort.
Our metric evaluates the duration (based on human expert time) of tasks that frontier AI systems can execute autonomously with a 50% success rate. The shaded region, demarcating a 95% confidence interval computed via a hierarchical bootstrap over diverse task families, underscores the robustness of our measure.
Introduction: Bridging AI Performance and Real-World Utility
Forecasting the evolution of AI technologies is vital for both harnessing their benefits and mitigating potential risks. While current frontier models demonstrate superhuman performance in tasks like text prediction and domain knowledge challenges, they still encounter difficulties when attempting to coordinate a series of actions over extended periods. This discrepancy between benchmark supremacy and practical application underscores the importance of our proposed metric: the ability to complete longer, human-timed tasks.
At a technical level, we analyze performance by fitting logistic curves to the success rate of each model across a spectrum of human-defined task durations. The point at which these curves intersect a fixed reliability threshold — for example, 50% — serves as an indicator of the model’s effective time horizon. Practically speaking, while models like Claude 3.7 Sonnet perform exceedingly well on tasks taking up to a few minutes, their reliability drops precipitously for tasks that require several hours of expert human time.
Technical Deep Dive: Methodology and Analysis
This study leverages a broad dataset of multi-step software and reasoning tasks. For each task, we record the time required by a human expert to complete it, which serves as a proxy for task complexity. A logistic regression model is then used to map the success probability of the AI against the duration metric. The analysis shows near-perfect success rates for tasks under 4 minutes and dramatically lower rates for tasks exceeding 4 hours. Such a trend robustly captures the limitations in current AI models when it comes to chaining complex steps despite their prowess in isolated challenges.
Recent experiments, including evaluations on subsets drawn from SWE-Bench Verified, have shown even faster doubling times – under 3 months. This indicates that methodological details, such as the operationalization of task time and the exclusion of code familiarization phases, can further refine our understanding of AI progress. Sensitivity analysis using 10,000 random perturbations confirmed that even large measurement uncertainties (up to a 10x divergence) would shift the forecast for achieving month-long autonomous performance by only about two years.
Furthermore, our analysis incorporated hierarchical bootstrapping over task families, individual tasks, and multiple attempts, ensuring that our computed 95% confidence intervals robustly capture variability and external noise. Emerging news in the field now suggests that similar trends could be observable in specialized tasks beyond software development, such as legal reasoning and advanced scientific discovery, indicating expansive applicability of our metric.
Methodological Insights: Robustness and Future Directions
Our approach is not merely a retrospective analysis of AI progress; it also opens pathways for more predictive benchmarking. By categorizing tasks based on human completion times, we can better align benchmark evaluations with real-world implications. For instance, companies developing remote executive assistance tools must now weigh the intelligence behind micro-decisions versus the ability to autonomously complete high-level project tasks.
We have further validated our approach through extensive sensitivity tests. Different task distributions – ranging from very short, routine tasks to a diverse collection known as HCAST and RE-Bench – consistently exhibit our identified exponential growth trend, with a clear signal across various levels of difficulty. This multifaceted approach provides a concrete link between state-of-the-art benchmarks and the actual capabilities that drive workplace automation.
Additional Perspectives: Expert Opinions and Technological Implications
Leading AI researchers and industry experts have weighed in on our methodology, noting that focusing on task length as a proxy for cognitive continuity could revolutionize how AI capability is quantified. Experts in AI policy argue that such an understanding will be crucial for risk management, as longer-duration tasks often involve multi-step decision-making processes that could impact system reliability and safety.
Moreover, technical innovators in the cloud and infrastructure sectors are excited by the potential to integrate these metrics into automated scaling systems. For example, dynamic resource allocation in cloud computing could leverage predictions from our metric to optimize workload distribution across vast data centers. As AI systems become more autonomous, the ability to forecast their task-handling limits will be instrumental in designing safeguards and robust failover mechanisms.
Future Forecast and Real-World Impact
The exponential trend observed over the past six years, with a doubling time of approximately 7 months, suggests that we are on the brink of a transformative phase in AI utility. If current development trajectories persist, we expect that within 2 to 4 years, AI agents will be able to autonomously execute projects that span entire weeks—introducing significant shifts in industries ranging from software engineering to strategic management.
The technical challenges of extending these capabilities are manifold. Beyond optimizing existing architectures, enhancements in memory management, context retention, and cross-modular reasoning will be key. Furthermore, as AI systems become capable of month-long tasks, ethical and operational risk management strategies become paramount. Regulatory bodies and industry leaders must collaborate to ensure that this leap in capability is paralleled by adequate safety protocols.
Community Collaboration and Opportunities for Contribution
We are excited to share our results with the broader community and encourage replication and extension of our work. Open-sourcing the infrastructure, data, and analysis code has been a crucial step in this direction. Researchers and practitioners are invited to utilize our GitHub repositories to explore novel task domains and refine the existing model evaluations.
In addition, METR is actively seeking innovative talent to further this research. Our multidisciplinary team is not only evaluating current benchmarks but also designing new ones that directly map to the promise and pitfalls of extended AI autonomy. Interested collaborators can review the listed roles on our careers page to join this forward-thinking initiative.
Conclusion
This work has significant implications for AI benchmarking, forecasting, and risk management. By measuring AI performance through the lens of task length—anchored to human expert completion times—we introduce a metric that captures both relative and absolute capabilities. Our analysis demonstrates a robust exponential trend in AI progress, suggesting that frontier systems will soon be able to autonomously manage month-long projects. Given the far-reaching benefits and associated risks, this approach offers a meaningful framework for both future AI evaluations and proactive governance.
As we continue to refine our methodologies and gather data from emerging research, such as the latest insights from specialized evaluations, the community’s collaboration will be essential in translating these technical advances into real-world improvements.
For further discussion, insights, and collaboration, please refer to our tweet thread and join the discourse on our community forums.