OpenAI’s GPT-Powered Spam Campaign: Unveiling the Technical and Security Challenges
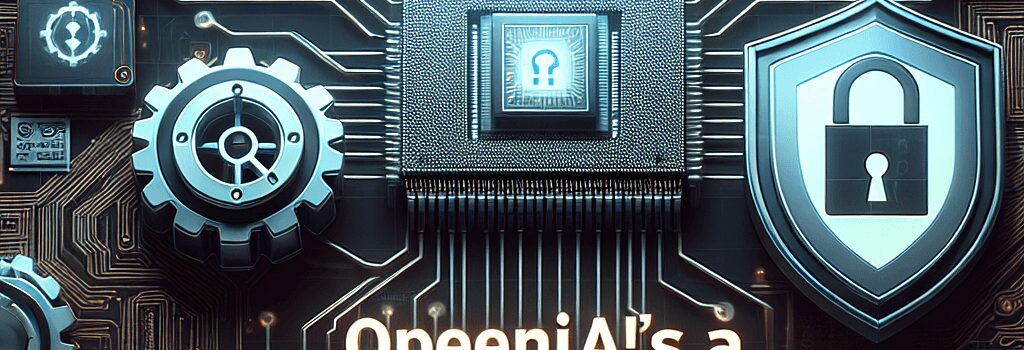
Recent findings from security researchers have revealed that spammers have harnessed the power of OpenAI’s GPT technology to launch a sophisticated spam campaign, successfully bypassing conventional filters. Over a four-month period, this campaign generated a massive blast of more than 80,000 unique messages targeting small- and medium-sized websites. This incident not only underscores the dual-use dilemma of large language models, but it also illuminates the evolving landscape of threats in AI-powered cyberattacks.
Overview of the Attack
According to the report published by SentinelOne’s SentinelLabs, spammers deployed a framework known as AkiraBot to automate and personalize marketing spam messages. Unlike traditional spam—which often relies on uniform content—these messages were individually tailored using OpenAI’s chat API, specifically a variant of the GPT-4 model labeled as gpt-4o-mini. By replacing variables such as website names dynamically at runtime, each recipient received a message crafted to appear as if it were uniquely composed for them. This level of customization allowed the emails to cleverly dodge spam-detection filters that typically look for similarity across bulk-distributed messages.
How AkiraBot Exploited GPT Technology
The AkiraBot framework, built on Python-based scripts, was designed to rotate various domain names advertised within the messages. This rotation, combined with the automation of the messaging process, created an environment where the GPT model could be seamlessly integrated. The chatbot was set into action by first receiving a prompt resembling: “You are a helpful assistant that generates marketing messages.” The command instructed the AI to incorporate the recipient’s website name into a brief marketing pitch, making each message appear contextually relevant.
This technique of leveraging GPT technology for personalized message creation is technically significant. It demonstrates how prompt engineering can be used to effectively bypass heuristic algorithms in spam filters. By generating near-unique content on each iteration, the underlying natural language generation capabilities of GPT-4 help defeat filters that rely on pattern detection and duplication across mass communications.
Technical Analysis: Under the Hood of the Spam Operation
Detailed log files obtained by SentinelLabs reveal that the operation was both scalable and resilient. The logs show successful delivery of spam messages to over 80,000 websites between September 2024 and January 2025, while roughly 11,000 target domains registered failed deliveries. This high success rate is bolstered by multiple factors:
- Dynamic Content Generation: The use of a large language model allows for diverse phrasing and syntax, reducing the likelihood of triggering spam filters.
- Domain Rotation: By continuously changing the advertised domains, the bot avoided static signatures that could be flagged by automated systems.
- Automated Scripts: Python-based scripts managed the timing, distribution, and personalization of each message, showcasing a high degree of automation and precision targeting.
Security Implications and Expert Opinions
Security experts have voiced concerns over the unintended consequences of making powerful AI tools widely accessible. The ease with which the GPT model was repurposed for spam tasks is a testament to the challenges of regulating AI-driven platforms. Alex Delamotte and Jim Walter from SentinelLabs emphasized that the diverse and customized outputs generated by the model render traditional spam filtering methods less effective.
Dr. Helena Morris, a cybersecurity researcher specializing in AI threats, commented, “The use of AI for generating bespoke spam messages represents a significant escalation in cyber threat complexity. It forces us to rethink our filtering strategies and invest in more adaptive, behavior-based anomaly detection systems.” Experts argue that proactive monitoring and a comprehensive understanding of AI behavior in production environments are essential for mitigating such threats.
Broader Implications for AI and Cybersecurity
This episode shines a light on the broader implications of deploying large language models in public domains. While AI offers a plethora of applications from customer support to content creation, it also presents significant risks when misused. The incident has raised questions about the balance between innovation and security, especially when considering that enforcement mechanisms can be largely reactive. OpenAI’s subsequent revocation of the spammers’ account serves as a reminder that policy framework and monitoring need to evolve in tandem with technology.
Mitigation Strategies and Future Outlook
To address these emerging threats, both AI developers and cybersecurity professionals must work in tandem. Improved AI auditing practices, enhanced real-time monitoring of API usage, and stricter adherence to usage policies are critical steps towards more secure implementations. Additionally, the development of advanced machine learning models that can detect subtly varied spam messages in real time is a promising avenue for future research.
Looking ahead, the security community is actively exploring how to best leverage AI to defend against AI-driven attacks. Collaborative efforts between cloud service providers, API developers, and cybersecurity firms will be essential in building robust defenses to prevent abuse of these powerful tools.
Conclusion
The AkiraBot spam campaign is a stark example of how cutting-edge AI technology can be exploited for malicious purposes. It illustrates the pressing need for enhanced detection methods and proactive security policies. As large language models continue to advance, the interplay between technological innovation and cybersecurity will remain at the forefront of digital security discussions.
OpenAI, along with other industry leaders, faces the ongoing challenge of balancing accessibility and functionality with robust safeguards against misuse.