Copyright Office and AI Training: Implications Ahead
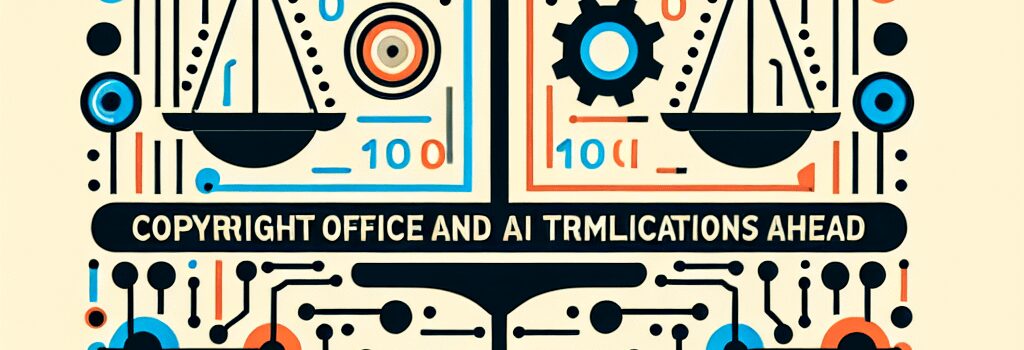
Background: A Sudden Power Shift
On May 8, 2025, President Donald Trump abruptly dismissed Librarian of Congress Carla Hayden and U.S. Copyright Office Chief Shira Perlmutter, mere days after Perlmutter’s office published a pre-publication report challenging the sweeping fair use claims of major AI developers. The move triggered condemnation from members of Congress, publisher and author groups, and open-source advocates, who decried the firings as an unprecedented executive intrusion into an independent cultural institution.
Key Findings of the Pre-Publication Report
The Copyright Office’s draft report evaluated more than 10,000 public comments on whether training large-scale generative AI models using copyrighted works constitutes fair use under U.S. law (17 U.S.C. § 107). It emphasized two factors as most determinative in future litigation:
- Factor 1: Purpose and character of use (whether the use is “transformative,” adding new expression or meaning).
- Factor 4: Effect on the potential market (whether AI outputs supplant or undercut creative markets for original works).
Notable conclusions included:
- “Unlicensed harvesting of copyrighted books, articles, and multimedia for model pre-training may infringe where outputs replicate or compete with the original.”
- A “consent framework” is needed, shifting the burden from authors opting out to developers securing explicit licenses.
- Training on pirated or paywalled datasets weighs heavily against fair use, even if not dispositive.
- Some transformative uses—such as summarization tools, grammar correction, and domain-specific embedding services—remain squarely within fair use.
Standoff at the Library: Capitol Police and Intruders
Days after the dismissals, social media buzzed with reports of a standoff at the Copyright Office entry in the Library of Congress between Capitol Police and two men claiming to be the new Deputy Librarian and Acting Copyright Director. Confidential sources later identified them as Brian Nieves and Paul Perkins; their true authority remains unverified. Capitol Police confirmed no forcible removal occurred, but the incident underscored the institutional chaos following the firings.
Technical Analysis of AI Training Data Requirements
Large language models (LLMs) and foundation models rely on multi-stage training pipelines:
- Data collection: Crawling & scraping web pages, books, code and articles. Typical corpora exceed 1–5 trillion tokens.
- Preprocessing & tokenization: Converting text to byte-pair encoded tokens or WordPiece embeddings to feed into Transformer architectures.
- Pre-training: Self-supervised objectives (masked-language modeling, next token prediction) on diverse datasets for several weeks on GPU/TPU clusters.
- Fine-tuning / RAG: Supervised or retrieval-augmented generation for domain adaptation (e.g., legal drafting, code synthesis).
Experts note that while some copyrighted content is necessary to capture linguistic nuance, the exact volume required for a “viable” model remains unsettled. Dr. Emily Bender (University of Washington) told us: “We don’t yet know the minimum viable dataset for competitive performance; wholesale ingestion of every text might be overkill and legally risky.”
Licensing Frameworks and Platform Solutions
Industry trade groups and legal scholars are exploring technical and contractual pathways to compliance:
- Token-based licensing: Metering API calls and assigning royalty rates per million tokens generated from proprietary corpora.
- Data marketplaces: Decentralized platforms using smart contracts (on Ethereum testnets) to automate royalty distribution to authors.
- Federated training: On-device or edge training that uses local user content under user consent, minimizing centralized bulk licensing.
According to Marketa Trimble of the Stanford Copyright, Open Science, and Data Access (CODA) Lab, “Layer 2 licensing solutions on public blockchains could ensure transparency, immutability of deals, and prompt payments at scale.”
Legal Precedents and Court Alignment
Emerging case law appears to echo the Office’s guidance. In Authors Guild v. OpenAI, filed earlier this year, U.S. District Judge Vince Chhabria pressed Meta on its use of a pirated books dataset, remarking: “I don’t see how reproducing millions of copyrighted pages can be transformative when the model churns out substitutes for your own work.”
Industry stakeholders note that only courts can definitively adjudicate fair use, but many see judicial trends favoring tighter scrutiny of unlicensed datasets:
- Factor 1 debates hinge on whether generative outputs add “new expression or meaning” rather than merely reformatting existing texts.
- Factor 4 analyses are likely to consider lost subscriptions or licensing fees attributable to AI-generated substitutes.
Expert Opinions and Industry Backlash
Reactions to the report and subsequent firings have fallen along predictable fault lines:
- Tech industry: The Computer & Communications Industry Association warned that an expansive view of market harm could allow rights holders to block any usage with hypothetical effects on ancillary markets.
- Civil liberties advocates: Free Speech & Free Press groups caution that onerous licensing could chill open-source innovation and restrict academic research.
- Creators’ coalitions: Author and publisher alliances have lauded the Office’s stance, emphasizing fair compensation for creative labor.
“Our goal isn’t to strangle AI development,” says Courtney Radsch of the Open Markets Institute, “but to ensure that creators aren’t bypassed when their works fuel these multibillion-dollar systems.”
International Context: EU and UK Developments
Across the Atlantic, the European Union’s AI Act and the UK’s Digital Markets, Competition and Consumer Bill each include provisions on copyright transparency and data traceability. Notably, the EU recently mandated “litigation support logs” for high-risk AI systems, requiring vendors to document training sources and usage footprints.
Global research hubs are already experimenting with “synthetic data” pipelines, generating AI-crafted substitutes to reduce reliance on copyrighted inputs. Early results show up to a 15% drop in downstream model performance, underscoring the trade-off between legal safety and linguistic richness.
Future Outlook and Congressional Action
Senate and House committees have rushed to draft new AI copyright legislation. Proposed bills include:
- Mandatory AI licensing registries: Central federal databases where developers register data sources and pay tiered fees.
- Safe harbor carve-outs: For nonprofit, educational, and low-revenue research uses.
- Revenue-share mandates: Requiring 2–5% of commercial AI revenues to be pooled for collective author compensation.
The next six months will be critical. If the White House sustains its pushback against the Copyright Office’s report, newly nominated leaders could reshape the guidance or slow its finalization. Simultaneously, courts will begin ruling on high-profile fair use suits, potentially setting binding precedents ahead of any statutory fix.
Conclusion
The abrupt firing of Shira Perlmutter—and the rapid political fallout—underscores how copyright policy now sits at the crossroads of technology, business, and culture. As AI systems grow more capable, the question of how they access, transform, and monetize creative works will remain one of the most consequential debates of this decade.