AI Use and Its Impact on Professional Reputations
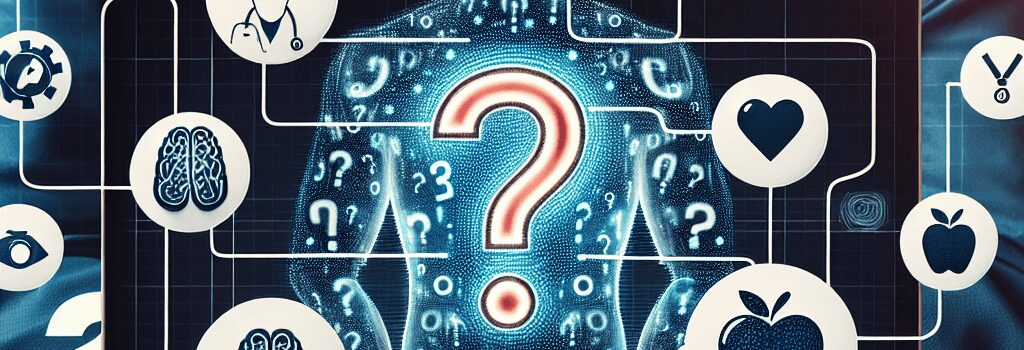
New research from Duke University sheds light on an unexpected downside of integrating AI tools in the workplace: social stigma. Published in the Proceedings of the National Academy of Sciences (PNAS) in May 2025, the paper “Evidence of a social evaluation penalty for using AI” investigates how colleagues and managers perceive employees who rely on generative AI platforms such as ChatGPT, Claude, and Gemini.
Key Findings from the Duke Experiments
Researchers Jessica A. Reif, Richard P. Larrick, and Jack B. Soll presented four controlled experiments involving over 4,400 participants. Their core observations included:
- AI users were consistently rated as 20–30% more lazy and less competent than peers using traditional software.
- Non-AI-using managers in a hiring simulation were 30% less likely to select AI-fluent candidates, whereas AI-savvy managers preferred them by 25%.
- Demographics such as age, gender, and role did not moderate the effect, suggesting a universal bias across professions.
- Disclosure reluctance was high: 62% of participants admitted they would hide AI use from supervisors to avoid judgment.
The Technical Mechanics of AI Assistance
Contemporary generative AI systems are built on transformer architectures with self-attention layers and feedforward networks. Models like GPT-4 Turbo (with 175 billion parameters) and Anthropic’s Claude 3 Sonnet (over 1 trillion parameters across ensemble nodes) perform tasks ranging from code synthesis to legal brief drafting. Key technical considerations include:
- Latency and Throughput: Enterprise APIs often balance token processing rates of 200–400 tokens/sec with end-to-end response times under 2 seconds.
- Data Security: Deployments on Azure OpenAI Service or AWS Bedrock leverage encryption at rest (AES-256) and in transit (TLS 1.3), plus customer-managed keys for compliance.
- Prompt Engineering: Techniques such as zero-shot and few-shot learning optimize context windows and reduce overall compute costs by up to 40%.
Latest Industry Trends
Despite individual reluctance, enterprises are accelerating AI adoption. Microsoft recently reported over 100 million monthly active users for Copilot for Business, while Google’s Gemini AI now integrates real-time data pipelines in Vertex AI Workbench. However, a Gartner poll from April 2025 indicates that 56% of employees fear performance reviews might penalize AI reliance.
Regulatory frameworks are also evolving. The EU AI Act, now in its final trilogue stage, will require explicit disclosure when AI-generated outputs influence hiring, lending, or legal advice. NIST’s AI Risk Management Framework v2.0 recommends periodic bias audits, red-team testing, and human-in-the-loop oversight to ensure trustworthiness.
Comparative Historical Perspectives
Technological stigma is not unprecedented. In ancient Greece, Plato criticized writing as diminishing human memory. In the 1980s, calculators faced bans in classrooms amid fears they would erode arithmetic skills. When Lotus 1-2-3 arrived in the early PC era, some accountants viewed spreadsheets as tools for novices. Today’s AI stigma echoes these debates, illustrating a recurring pattern where labor-saving tools challenge established notions of expertise.
Mitigation Strategies for AI Stigma
To counteract negative perceptions, experts recommend:
- Transparent Attribution: Clearly label AI-generated sections in reports or presentations, using version control tags or metadata annotations.
- AI Literacy Programs: Offer workshops on prompt design, bias detection, and security best practices to position AI as a complement to human skill.
- Performance Frameworks: Incorporate AI competencies into KPIs, rewarding employees who optimize model use responsibly and ethically.
As AI pioneer Andrew Ng observes, organizations that treat AI fluency as a core competency gain a competitive edge by unlocking novel sources of innovation.
Organizational Policies and Psychological Impacts
Workplace psychologists note that adopting generative AI can trigger confirmation bias and stereotype threat. Employees may internalize negative expectations, leading to reduced self-confidence. Companies like Salesforce and IBM have piloted optional AI overlays—where suggestions appear in a side panel rather than in-line—to give users control over AI assistance.
Additionally, a 2025 study from the University of Copenhagen found 8.4% of AI deployments inadvertently increased workload by generating oversight tasks—verifying model outputs, correcting hallucinations, or auditing for compliance. Robust governance processes and continuous feedback loops are essential to avoid such hidden burdens.
Conclusion
The Duke University study illuminates a complex reality: while generative AI promises significant productivity gains, it also carries social costs that can undermine professional reputations. As enterprises push forward with AI initiatives, balancing technical integration with cultural acceptance will be critical. By establishing transparent policies, fostering AI literacy, and aligning incentives to reward responsible use, organizations can mitigate stigma and fully leverage the transformative potential of AI.