Protecting Human Creativity in the Age of AI
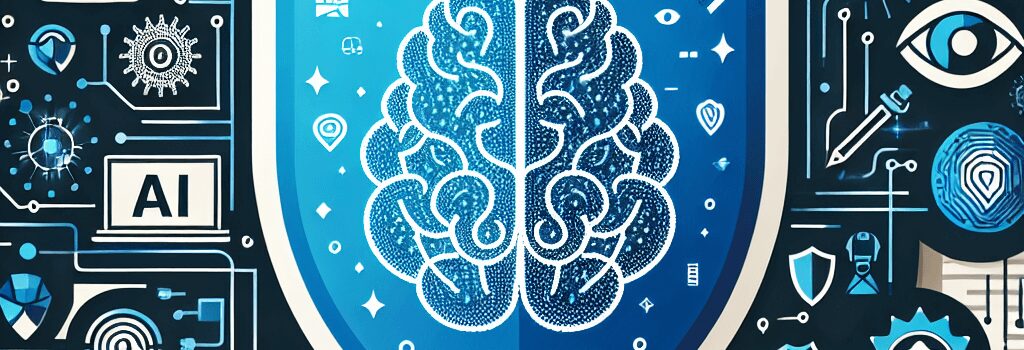
Ironically, our present AI era has cast a bright spotlight on the immense value of human creativity as breakthroughs in machine learning threaten to undermine it. As technology giants race to train ever-larger models—GPT-4 with its 175 billion parameters consuming over 800 GB of pretraining data, or Google’s PaLM with 540 billion parameters ingesting 780 TB of web text—these systems vacuum up creative works and subsequently spew synthetic media in staggering volume. That tidal wave risks drowning out the human spark in an ocean of algorithmic “pablum.”
A Limited Resource
Human creativity isn’t an industrial commodity. It’s produced by finite, embodied agents—biological brains that sleep, rest, and draw inspiration from lived experience. Each brain is effectively a 100 trillion–synapse neural network, generating novel idea combinations through associative processes that depend on emotion, context, and serendipity. In contrast, a trained large language model (LLM) simply performs statistical pattern matching across its 1.5 trillion–token training corpus. If every system refers to the same scraped dataset, we risk flattening cultural nuance into a mediocre mean.
AI’s Creative Debt and Training Data
Every AI writing assistant, image synthesizer, or music generator stands on a towering pile of human works. In 2024, a NeurIPS paper warned of “model collapse” when models are retrained on their own outputs, leading to an irreversible loss of informational fidelity—akin to repeatedly JPEG-compressing a photograph. Adobe’s Firefly, by contrast, was trained exclusively on licensed stock assets and public domain content, showing that more sustainable curation (albeit at higher licensing cost) is technically viable. Meanwhile, OpenAI and similar labs rely on broad web scraping under “fair use” claims, ingesting trillions of words without granular opt-in mechanisms.
Copyright as Crop Rotation
Copyright law was originally conceived as a resource-management tool—time-limited exclusivity that replenishes the public domain. Yet decades of legislative extensions have pushed the expiration cycle beyond 70 years in many jurisdictions, delaying regeneration. Now wholesale AI extraction further disrupts the balance between creators as consumers of ideas and creators as producers. In the U.S., purely AI-generated outputs cannot be copyrighted, potentially flooding the public sphere with derivative content devoid of true innovation.
The Creative Ecosystem Under Strain
Aggressive AI crawlers such as OpenAI’s GPTBot function like distributed denial-of-service attacks on smaller sites. Cloudflare’s telemetry shows bots making millions of requests per day, and Wikimedia reported a 50% surge in bandwidth use when crawlers ignored robots.txt directives—forcing the foundation to divert compute and operational budgets away from content curation. On the front end, Google Search has seen a 30% uptick in “spammy, low-quality” auto-generated pages, sometimes outranking original journalism—a form of digital pollution compared by Cambridge’s Ross Anderson to dumping plastic into the oceans.
Technical Safeguards Against AI Overreach
- Proof-of-Work Rate Limiting: Challenge-response protocols that require clients to solve crypto puzzles (e.g., Hashcash) before granting access, throttling industrial-scale crawlers without penalizing human users.
- Watermarking and Fingerprinting: Injecting imperceptible signals into text (e.g., bit-level modifications at a 1% noise ratio) or images (e.g., frequency-domain patterns) to allow automated detectors to distinguish AI output.
- Shared Blocklists and Robots Extensions: Initiatives like ai.robots.txt and Nepenthes tarpits slow down known bot fingerprints, while community-managed crawler registries help maintain fair-use boundaries.
- Data Provenance Frameworks: Standards such as W3C PROV and digital notarization (e.g., Ethereum smart-contract timestamps) can certify original human authorship and trace data lineage.
Economic and Licensing Frameworks
- Royalty Clearinghouses: Analogous to ASCAP or BMI for music, a proposed system could collect micropayments via blockchain (ERC-1155) when AI models use protected works, ensuring artists receive per-generation compensation.
- Opt-In/Opt-Out Registries: The EU AI Act’s Article 10 mandates transparency and consent for training data, while a global `.artists.txt` registry could let creators declare usage rights.
- Collective Management Societies: Proposals suggest empowering agencies to negotiate blanket licenses with AI labs, pooling fees from corporate subscribers to fund grants and fellowships for human creators.
Policy and Governance: Global Approaches
On the policy front, the EU’s AI Act (expected Q4 2025) classifies unconsented data scraping as a high-risk practice, requiring impact assessments and human oversight. UNESCO’s draft Recommendation on the Ethics of AI urges member states to balance innovation with cultural preservation. In the U.S., the Copyright Office’s 2024 ruling on “Thaler” author cases restricts registration for AI-only works, reinforcing that an identifiable human must be the creative agent.
Protecting the Human Spark
Unlike an LLM, a human artist grows over decades, learning from failed drafts, heartbreak, and triumph. While retrieval-augmented generation (RAG) pipelines can fetch fresh context—using vector stores like FAISS to pull recent news articles—they still depend on high-quality human-produced anchors. If the reservoir of genuine content is polluted, even the smartest RAG systems will degrade into echo chambers.
AI as Creative Support
Used responsibly, generative AI can accelerate workflows: Adobe’s Sensei neural filters prototype in under a second on an NVIDIA RTX 4090, and GitHub Copilot’s Codex engine suggests code snippets in real time, reducing boilerplate by up to 40%. As with the typewriter or the synthesizer, the tool amplifies human intent—empowering the skilled practitioner to explore new ideation paths rather than replace them.
Cultivating the Future
A sustainable creative ecosystem demands technical standards, economic incentives, and enlightened policy. Watermarking protocols must become mandatory API requirements. Licensing fees could underwrite public-domain fellowships akin to Japan’s “Living National Treasures” program, preserving endangered art forms. Meanwhile, an “AI commons” model would declare any model trained on public data as a shared social resource, with its API revenues funneled back into grants and infrastructure.
Invest in People
While we tackle these systemic challenges, one strategy is immediate and profound: invest in human talent. Organizations that blend diverse human insight with thoughtful AI augmentation will outcompete those chasing indiscriminate automation. In this AI age, human creativity is our scarcest, most precious resource—one we must steward as carefully as any natural ecosystem.